RESOURCES
White Papers
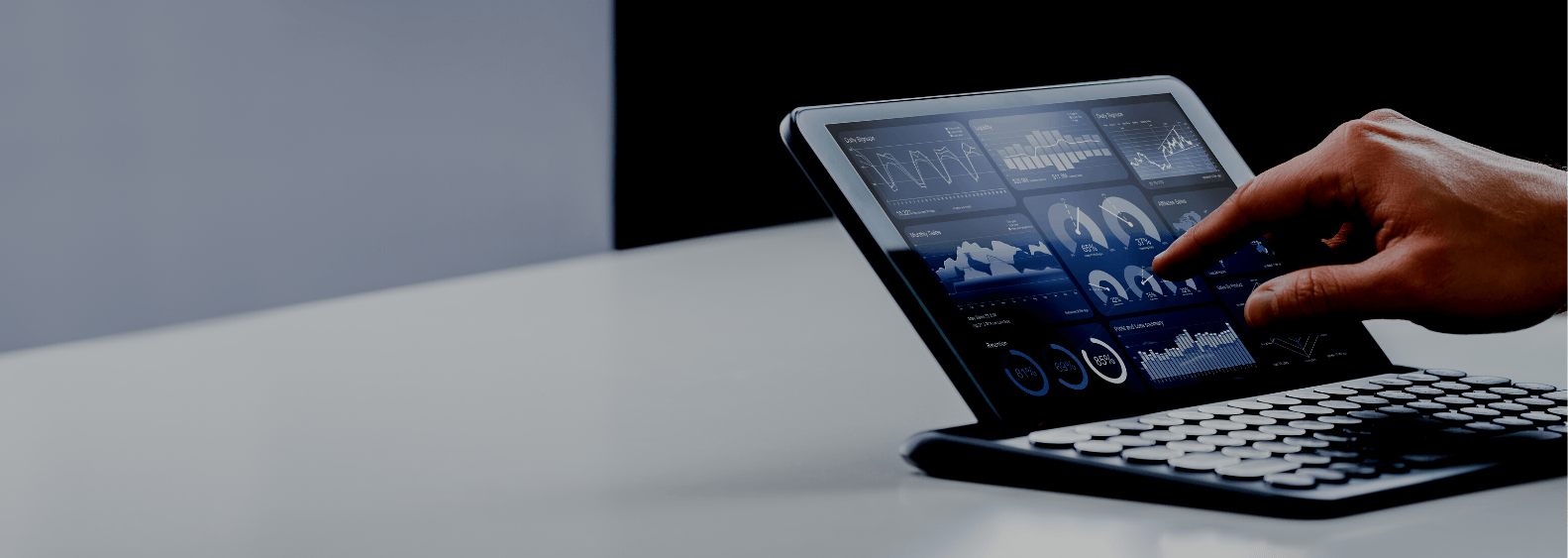
Abstract Ensuring optimal machine operation is of central importance in any industrial activity. Any degradation in operation, be it availability, performance, or quality, impacts the bottom line, which can be millions of dollars in high volume manufacturing. Therefore planned maintenance at regularly scheduled intervals is a broadly accepted concept and practice in the industry. However, such a practice is sub-optimal in serving the goal of optimal machine operation. Regularly scheduled maintenance can be overkill on the one hand, leading to unnecessary scheduled machine downtime and therefore productivity loss. On the other hand it doesn’t entirely prevent an unforeseen machine break down. The Holy Grail is to be able to predict when to schedule necessary maintenance under any circumstance before a break down occurs. Big data analytics and machine learning are the primary tools available to manufacturers to predict when to schedule maintenance. Maintenance Strategies Compared Maintenance strategies can be categorized as follows: Reactive maintenance. Maintenance is only performed when the machine breaks down. Preventive maintenance. Maintenance is performed at regular intervals. Conditional maintenance. Maintenance is scheduled in anticipation of a breakdown, based on monitoring of the asset and using prior experience to assess the need to schedule maintenance. Predictive maintenance. Maintenance is scheduled in anticipation of a breakdown, using big data analytics and machine learning to predict the probability of a failure by models trained on discerning usage and wear data patterns. Since maintenance is scheduled not later and not sooner, but at the right moment, predictive maintenance can also be called just-in-time maintenance.
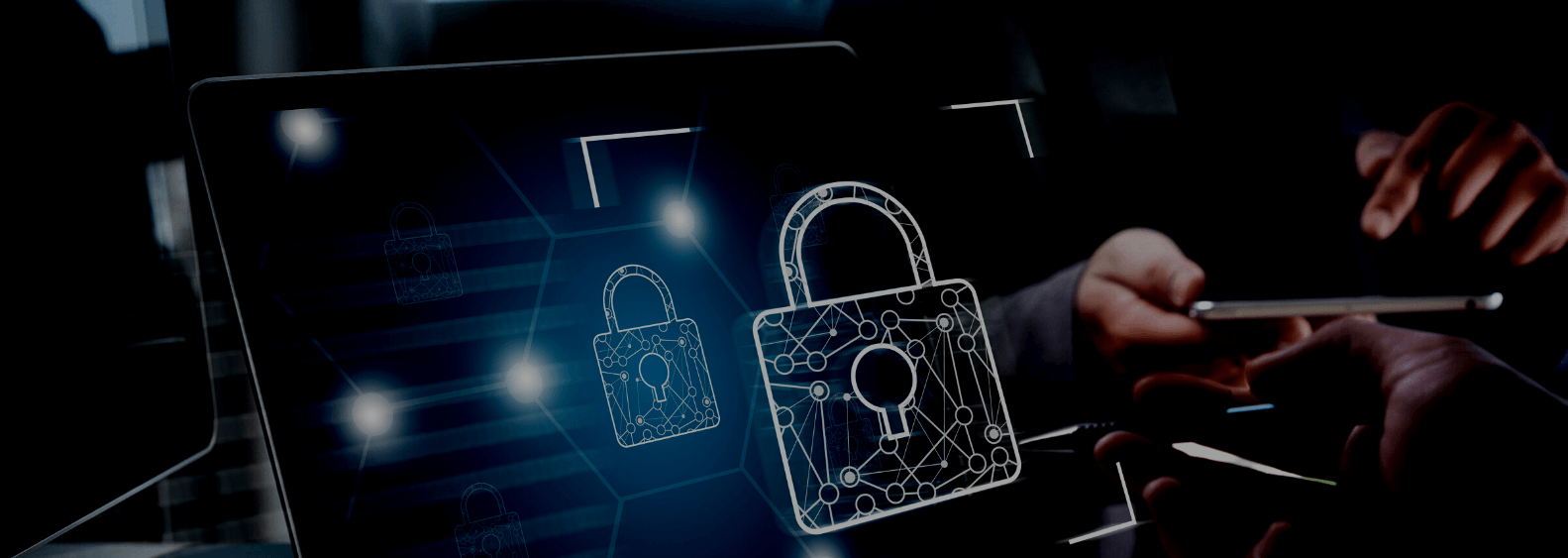
Introduction Although there are many parts of a factory that contribute to the overall effectiveness of a manufacturing operation, there is no doubt that what happens on the production floor greatly impacts overall effectiveness. Although control and operation of machines in a modern manufacturing operation is highly automated, many such operations rely on a mix of automation and manual operation for aspects that still require operator attention and intervention. Therefore, when measuring equipment OEE (overall equipment effectiveness) from availability, performance and quality, it is not only logical, but necessary to consider the operator as part of the machine. As such the Cyber-Physical System (CPS) or Digital Twin representation of the equipment must include the human-operator-in-the-loop (HOITL) so that operator data is included as part of the automated collection of data for the entire machine. The challenge is to do this in an unobtrusive fashion, so that the operator is not hampered in any way when interacting with the equipment. Therefore, use of a wearable device requires transmitting operator data wirelessly. An additional requirement is that the solution must be cost-effective. RFID was designed to meet all these three requirements. In this white paper we explore the use of this technology with GEMBO Precare agents that turn any machine into a CPS by streaming data in real-time to the GEMBO Precare Industry 4.0 IIoT platform. RFID Overview Industrial and commercial use power generators are complex systems, which are required to operate reliably without interruption and to be available whenever the need arises for backup power. Like with any complex system any number of things can go wrong and will go wrong, no matter the level of planning and preparation. The challenge is therefore to minimize the financial, operational and psychological cost of any malfunction or suboptimal functioning of the system. The following equipment related events negatively impact operational cost and customer satisfaction: Equipment breakdown. Equipment downtime, regardless of maintenance, repair, out of fuel or other reasons. Reduced power output. What if you could minimize the probability of any of these events and hence reap the fruits of reduced OpEx and increased customer satisfaction? The GEMBO PRECARE Solution RFID is an acronym for Radio Frequency IDentification. It is intended as a low power means of tagging animate and inanimate objects for identification via a wireless reader. Information about the object is stored in an RFID tag’s on-board Flash memory. Based on how the tag is powered, RFID tags are classified in two main categories: Passive RFID tags Active RFID tags. Passive RFID tags operate without the use of a battery. The tag uses its antenna as an induction coupling device to transfer power emitted via a reader’s antenna to power itself so that it can transmit its data to the RFID reader. The main components of a passive RFID tag are Rx/Tx antenna; Modem (modulator/demodulator); Codec (encoder/decoder); Logic control circuit; Memory; RF Energy harvester for passive tags, or battery for active tags. The main standards bodies for RFID are: ISO/IEC; IEEE; GS1/EPCglobal.
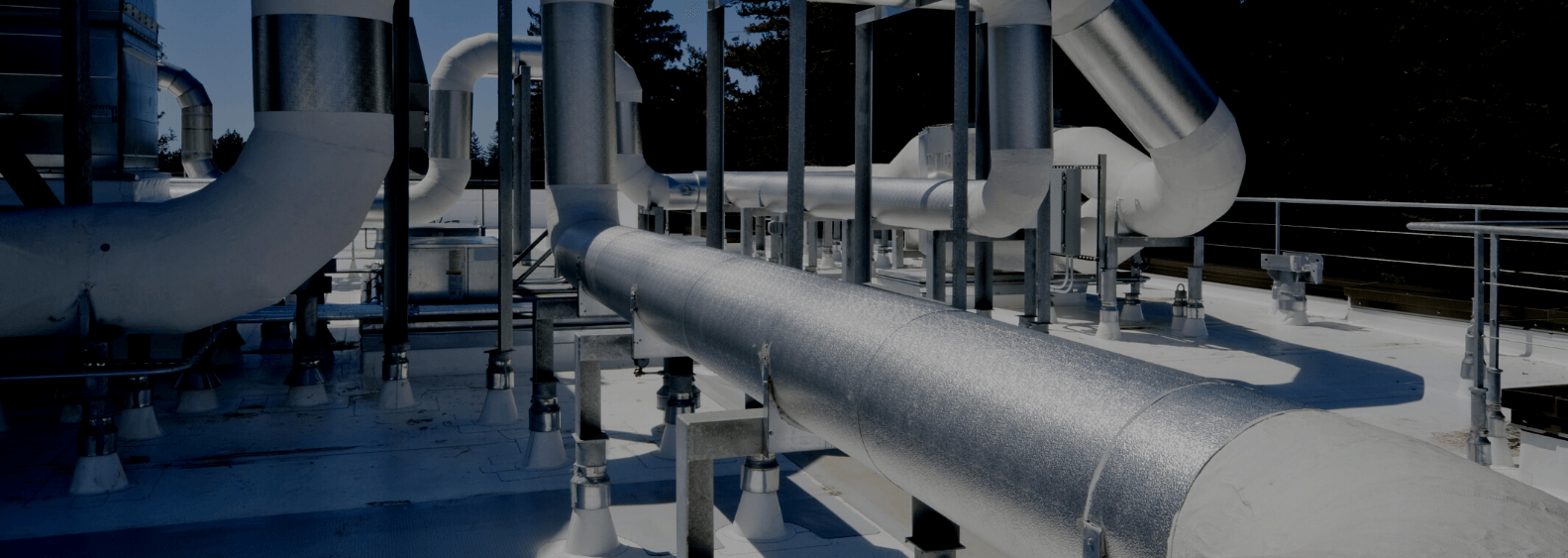
Abstract Customers of industrial and commercial use HVAC systems place high expectations on the quality, performance and availability of these systems. They expect them to perform at configured ambient settings with little or no interruption. The same OEE KPI and predictive maintenance principles used in the manufacturing industry apply to industrial and commercial use HVAC systems. With GEMBO Precare, HVAC OEMs and their service partners can achieve significant reductions in operational expenses and see significant increases in customer satisfaction. Negative Impacts on OPEX and Customer Satisfaction Industrial and commercial use HVAC systems are complex systems which serve users that just want them to work at their ambient preferences without interruption. Like with any complex system any number of things can go wrong and will go wrong, no matter the level of planning and preparation. The challenge is therefore to minimize the financial and psychological cost of any malfunction or suboptimal functioning of the system. The following equipment related events negatively impact operational cost and customer satisfaction: Equipment breakdown. Equipment downtime, regardless of maintenance, repair, out of fuel or other reasons. Reduced power output. What if you could minimize the probability of any of these events and hence reap the fruits of reduced OpEx and increased customer satisfaction? The GEMBO PRECARE Solution GEMBO Precare uses the power of data, analytics and machine learning to mitigate and minimize the impact of equipment related adverse events through the following: Predictive maintenance . Avoid costly unscheduled and unnecessary scheduled downtime. Instead determine when precisely to schedule maintenance. Catastrophic failure prevention . Avoid costly catastrophic failures, for instance by early detection of abnormal vibrations in rotating parts. Maintenance/repair time reduction . Avoid expensive maintenance/repair crew hours with prior knowledge of the kind of maintenance or repair and which spare parts are needed. Truck-roll reduction . Avoid unnecessary truck rolls by minimizing planned and unplanned downtime while simultaneously having sufficient information upfront to determine what needs maintenance and/or repair. Overall Equipment Effectiveness & customer satisfaction optimization . Maximize OEE and customer satisfaction by eliminating unnecessary and unplanned downtime by keeping any downtime at a minimum and by keeping customer premise equipment running at optimal performance. Predictive Maintenance GEMBO Precare collects failure data and uses machine learning to construct models that can accurately predict when a failure has the highest probability of happening. An example is shown below.
Case Studies
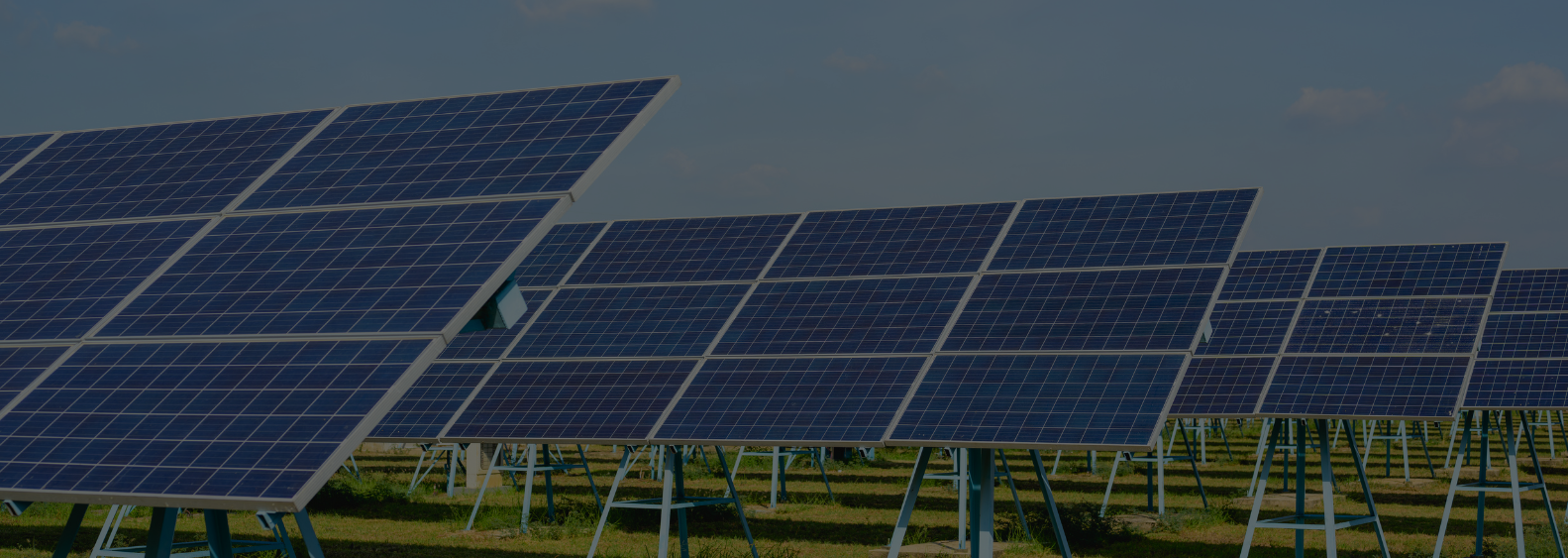
GEMBO ( www.gembo.co ) has a SaaS Industrial IOT Platform which provides enterprises and users with mission critical data, insights and decision making tools enabling cost reduction and revenue growth through optimization via the use of IoT, Artificial Intelligence and Machine Learning. Farm Operator Intro A large-scale solar farm operator, managing a 50 MW solar farm with significant investments in infrastructure. The solar farm has a current efficiency of 24% and a target of increasing this efficiency over time. The farm operates in an area where electricity prices are approximately $32 per MWh, and it has a large operational budget dedicated to maintaining its equipment. GEM has deployed the Precare cloud, predictive maintenance and solar power prediction Package, on the customers Data, extracted from the solar farm's SCADA system. Problem Due to the complexity of solar equipment and environmental factors, the customer has faced a gradual decline in efficiency, dropping from 26% to 24% over the past few years. As a result, the farm's revenue has been impacted by lower power output, and operational expenses have been steadily increasing. Furthermore, the lack of predictive maintenance (PdM) has led to frequent downtime and unplanned maintenance, further increasing the inefficiencies. The farm owner reached out for a solution that could improve operational efficiency, reduce costs, and enhance overall power production by using predictive analytics to forecast and mitigate potential issues before they occur. Solution After evaluating the farm’s needs and existing system, GEM’s Predictive Maintenance (PdM) and Solar Power Prediction packages were discussed and found as the right fit. This solution was implemented through GEM’s Precare analytics platform, which helps monitor and predict performance, detect faults early, and optimize maintenance schedules. The key components of GEM’s solution included: Real-time Monitoring: Continuous monitoring of solar inverters, panels, and environmental conditions. Predictive Maintenance: Advanced machine learning models to predict the likelihood of system failures and performance degradation. Power Output Forecasting: Predictive analytics to model expected energy production based on historical data and real-time inputs, leading to more accurate forecasting and optimizing grid management. Results After deploying GEM’s predictive maintenance and power optimization system, GEM provided the customer a calculation of a 3 year business analysis Financial Benefits: Revenue Increase: The system’s efficiency would increase by an additional $864,320 in revenue over 3 years due to the increased power output from the higher efficiency (25% vs. 24%). OPEX Reduction: Predictive maintenance would reduce the monthly operational expenses by 3%, leading to OPEX savings of $78,810 over the 3-year period. Net Benefits: The customer will realize a total net benefit of $758,130 after deducting the initial system cost and subscription fees. ROI: The ROI of 658.13% was computed from the net benefits of $758,130 after accounting for system cost investments.
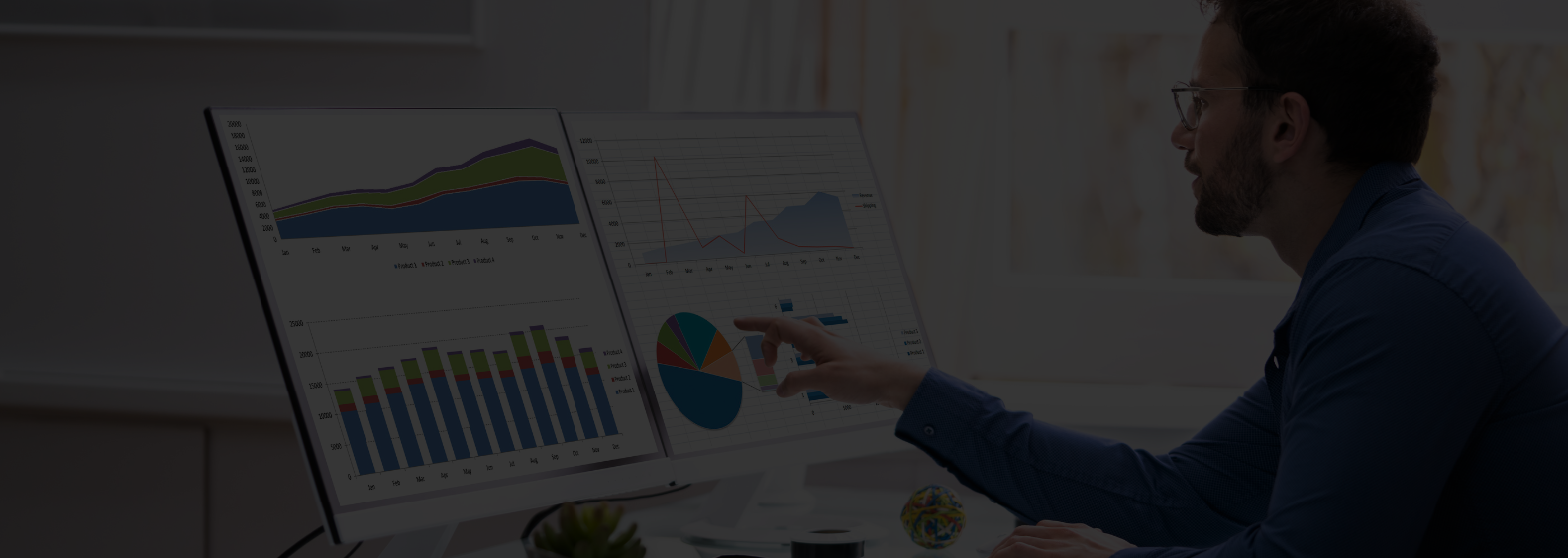
SELF SERVICE DATA STUDIO CASE STUDY This case study showcases GEMBO, a leader in SaaS Industrial IoT Platforms, enhancing a global manufacturer's operations by deploying its Precare Data Studio BI product across 70 machines. The solution, focused on self-service data management and analytics, resulted in significant manpower savings, improved self-service efficiency, and automated analytics distribution. Unique to GEMBO Precare is its ability to deploy independent sensors, connecting any machine to its cloud, thereby offering predictive maintenance and operational insights without substantial capital expenditure, distinguishing it from other market solutions.
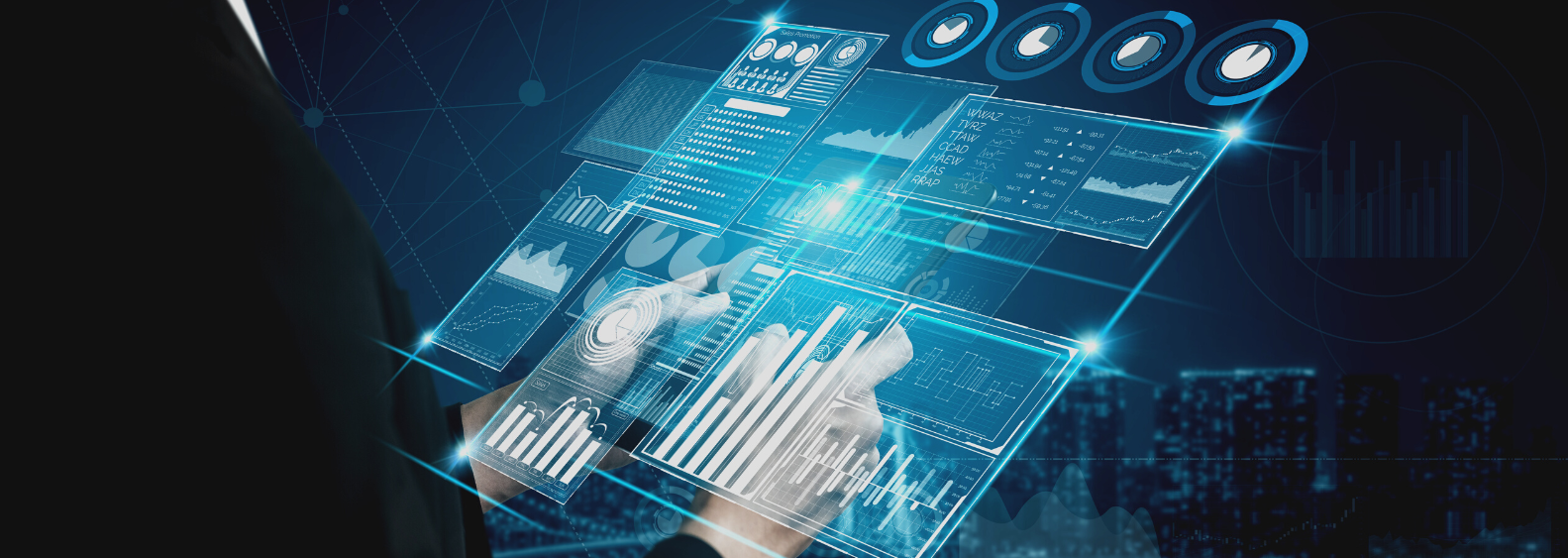
GEMBO ( www.gembo.co ) is a leading innovator in the world of SaaS Industrial IoT Platforms, with a proven track record of delivering cutting-edge solutions that help customers achieve their business goals. The company's platform uses a combination of IoT, machine learning, and AI to provide customers with real-time insights into their operations, enabling them to make better decisions and optimize their performance. GEMBO is committed to continuous innovation, and the company is constantly looking for new ways to use technology to help its customers succeed. Customer Intro The customer is a multi $B Tier one manufacturer, with multiple factories around the globe. Manufacturing lines vary between electronic manufacturing to semiconductor, serving a variety of markets including Automotive, Networking, Industrial, Audio and Gaming. GEM has deployed Precare Cloud , Precare Edge , OEE Availability and predictive analytics Package . Its footprint grew from a few machines to over 90 machines in a few of their factories. Problem The customer has been utilizing OEE Availability analytics to identify machines with low availability and assigning them to responsible technicians. The customer's management team has been overwhelmed with emails from technicians providing status updates on the machines after they have taken corrective actions. The customer intends to streamline the process of monitoring machine status from the receipt of OEE Availability status to the resolution of availability issues, enabling the machines to operate efficiently based on established availability KPIs. The customer has provided the following workflow to illustrate their requirements.
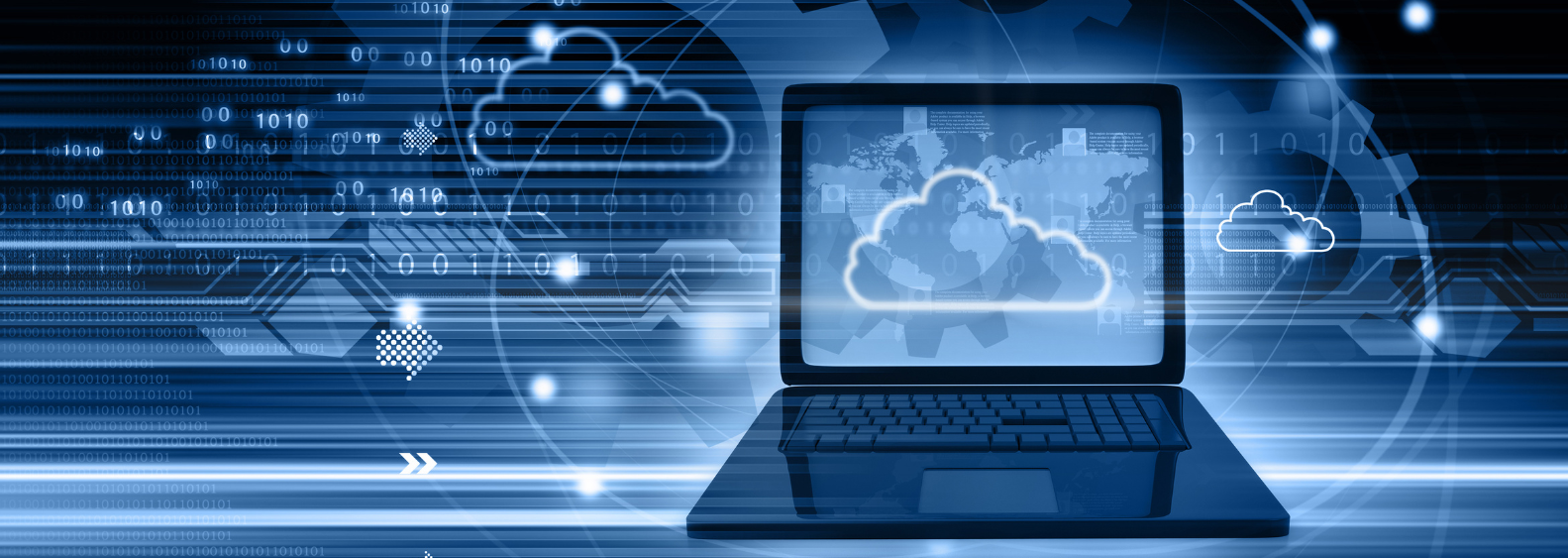
GEMBO ( www.gembo.co ) is a leading innovator in the world of SaaS Industrial IoT Platforms, with a proven track record of delivering cutting-edge solutions that help customers achieve their business goals. The company's platform uses a combination of IoT, machine learning, and AI to provide customers with real-time insights into their operations, enabling them to make better decisions and optimize their performance. GEMBO is committed to continuous innovation, and the company is constantly looking for new ways to use technology to help its customers succeed. Customer Intro The customer is a Tier 1 multi-billion dollar global manufacturing leader in the power, discrete semiconductor, and passive electronic components (PES) space. GEMBO has deployed a suite of innovative, cloud-based solutions to the customer, including Precare Cloud, Precare Edge, OEE Availability, Performance, and Quality packages. These solutions have been deployed to 70 machines across two factory floors, and the customer intends to scale the deployment across their entire Asia-Pacific footprint. Problem The customer's equipment engineering team has been managing the productivity of their machines through manual Excel reports. These reports are prepared by machine operators, checked and consolidated by supervisors, and then reported to management by factory floor managers. The customer needs to minimize or eliminate these manual reports to improve the accuracy of the reports and save on manpower costs. The following are examples of manual reports that the customer needs to eliminate: 1. Calculation of the share of subcategories of Performance and Quality 2. Calculation of the percentage of Planned Downtime to Total Run Time 3. Graphical presentation of OEE Availability with Time Frame These reports are time-consuming and error-prone. They also require a significant amount of manpower to create and maintain. By eliminating these manual reports, the customer can improve the accuracy of their productivity data and save on manpower costs. Solution After a technical discussion with the customer, GEM integrated the manual Excel reports into the OEE analytics framework. The team then implemented the following process: Define the customer requirements Gather the excel reports Create an output image showing changes in the analytics Define data to be collected Collect data from the customers Collect the data from customer Validate the completeness of the data based on the requirements Collect missing data Confirm with customer if all data are accurate and structured properly based on their requirements Prepare the design Overview Customer Requirements Use Case System Diagram Code Diagram Review and approval of the design Testing on Developer Environment Deployment to Production Customer acceptance Results The migration of previously manually prepared OEE reports to a digital format using OEE Analytics resulted in the following benefits: 100% savings on manpower costs, as the reports are now generated automatically and do not require manual input. 100% accuracy, as the data is collected directly from the machines and is not subject to human error. 100% time savings for management, as they no longer need to spend time reviewing and validating the reports. Improved decision-making, as the Operations Group can now access real-time data and insights to make better decisions. Increased efficiency, as the Operations Group can now focus on core tasks and not on data entry and reporting. Conclusions GEMBO Precare has a set of powerful data acquisition, analytics, predictive and OEE tools for manufacturing equipment. GEMBO Precare compiles critical KPIs that can be used to easily trace back which machine or machine subsystem is responsible for a low KPI score. GEMBO Precare is also able to make predictions at equal or better than humanly possible for machine maintenance to be scheduled before a failure occurs. But most importantly, unlike other market solutions, GEMBO Precare is able to deploy its own sensors independently from any machine controller and fully connect any machine data island to the GEMBO Precare Cloud at a fraction of the cost of a new machine; hence, saving manufacturers from having to make large and risky CAPEX and OPEX investments for new machines. Contact Us Visit us at www.gembo.co or contact us via email at sales@gembo.co
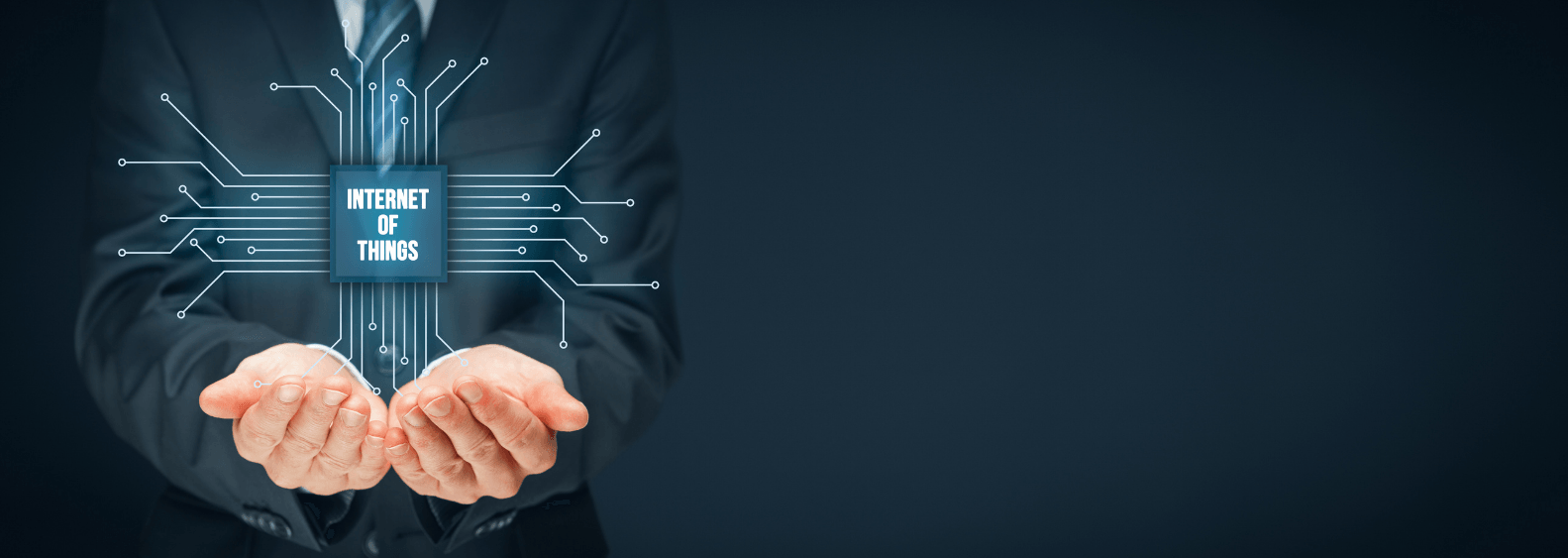
INTRODUCTION The GEMBO PRECARE platform enables manufacturers to seamlessly make the transition to Industry 4.0 literally overnight, while keeping their investments in legacy machines; thus, being able to tap into the potential of real-time access to machine data in order to improve efficiency, productivity and quality. Real-time access to machine data can be through any combination of sensors, serial and parallel port interfaces, Ethernet and sensors, no matter where the data is originating from, i.e., directly from the machine, or indirectly from a PLC or a data store on a network. Complex event process control at the edge of the cloud or at the machine itself requires hardware platforms with adequate processing power. GEMBO Precare supports edge processing through the use of powerful software agents. These agents take full advantage of the capabilities of the underlying hardware. In this white paper we show how the GEMBO Precare Industry 4.0 IoT platform takes advantage of Intel® x86 processor and Intel® Altera® Cyclone V SoC FPGA hardware capabilities to implement process monitoring and control at the edge in an industrial flow control application. GEMBO PRECARE MOTOR CONTROL AGENT Motors used in machines and production processes are very common and the ability to monitor them in real-time is understandably of crucial importance. GEMBO has developed an end-to-end motor control and monitoring solution in collaboration with Intel® and B&R Automation®, which takes advantage of the versatile and powerful GEMBO Agents for CEP at the edge and the GEMBO Precare cloud platform for monitoring and predictive maintenance. The process diagram shown below depicts a flow control application example. Control of a pump controls the flow rate in a pipe, and a safety valve prevents overpressure inside the pipe.
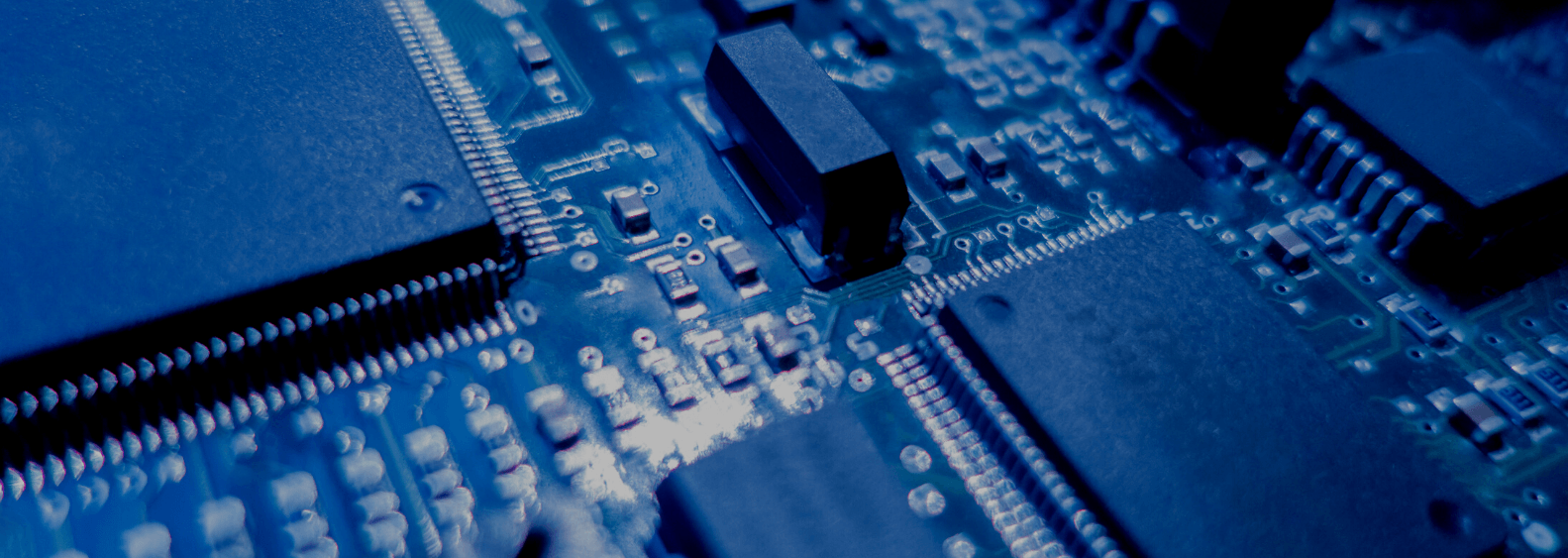
The Customer Semiconductor manufacturers are constantly under pressure to squeeze the most out of their capital and operational expenses, while at the same time facing tremendous pressure in a global market from their customers to deliver the highest quality within the shortest lead time. Whereas COOs are held responsible for CapEx, OpEx and overall performance across all manufacturing sites and business units, VPs at each business unit are responsible for CapEx, OpEx and overall performance of their own business unit, while factory floor directors are responsible for manufacturing operations at their plants to run as efficiently and productive as possible. In concrete terms this means minimizing unplanned machine downtime and maximizing machine throughput, while keeping manufacturing quality high; or in industry parlance, maximizing OEE (Overall Equipment Effectiveness). The Challenge Maximizing OEE in semiconductor manufacturing is a non-trivial task. The manufacturing workflow involves multiple stages; each handled by machines specialized for the particular operations involved in a stage. It is not uncommon to find machines from different OEMs handling the same or different tasks on the manufacturing floor, where some machines may be controlled by older generation Operating Systems (some with none), PLCs (Programmable Logic Controllers) that don’t have the ability to provide machine status, performance, quality and availability data in real-time to spot trends to predict when maintenance needs to be scheduled and optimize equipment effectiveness. This situation includes having no access at all to data for analysis and having access, but having to import the data in batch mode, missing crucial data. In addition, where the data is available, it is often in formats that differ from machine to machine, and more often than not, in formats incompatible with prevalent IIoT formats, such as JSON, XML, etc. To top it all off, a plethora of different communication protocols, ranging from SECS/GEMBO , SCADA and RS232/485 to various flavors of Industrial Ethernet compound the problem of access to data for analytics even further. As a consequence all stakeholders, from factory floor to corporate office, don’t have access to crucial data to help them make well-informed decisions towards OEE optimization in order to meet the end goal of growing bottom line revenue and market share. The Solution Industry 4.0 augments Industry 3.0 process control automation systems with big data and big data analytics. At one end of the Industry 4.0 spectrum this means real-time machine data access, while at the other end of the spectrum this means bridging the chasm between operational technology systems on the factory floor and information technology systems in the back-office. Industry 4.0 creates a digital twin or cyber physical system (CPS) of each machine on the factory floor. These digital twins allow all stakeholders to take advantage of the full Industry 4.0 spectrum of big data analytics opportunities. A 2018 PwC survey of the electronics manufacturing industry estimates that 45% of manufacturers are already deploying or are in process of deploying Industry 4.0 systems, while another 32% is planning to deploy Industry 4.0 systems within the next 5 years. The GEMBO PRECARE platform is able to offer semiconductor manufacturers a complete Industry 4.0 upgrade which is tailored to their specific needs, with all the benefits of big data analytics to optimize OEE, but without the cost of overhauling their existing machinery. The highlights of this solution are summarized below.