Heavy Industries Manufacturing Reaps Industry 4.0 Benefits with GEMBO Precare
CRITICAL KPIs AND PREDICTIVE ANALYTICS FOR ENHANCED OPERATIONAL
ABSTRACT
The advent of Industry 4.0 or smart manufacturing is transforming industries across the board from an automation-centered to a knowledge-centered focus. This is not surprising since the benefits of big data analytics for the purpose of making predictions and tracing the root cause of an issue is of extreme importance to any manufacturer, regardless of the industry sector. In this white paper we identify the unique problems that manufacturers in the heavy industries sector face in making the transition to smart manufacturing and explain how the GEMBO Precare IIoT platform solution helps solve these issues without the need for an overhaul of existing manufacturing infrastructure.
BENEFITS PERCEPTION OF INDUSTRY 4.0
A survey conducted by the Boston Consulting Group in 2016 on manufacturers’ expectations from Industry 4.0 shows that manufacturing cost as the top expectation gets the highest priority in the range of manufacturing cost to new revenue model, followed by product quality, while manufacturing cost scores the highest in expectation, followed by product quality.
This is not surprising since the main foundational element of Industry 4.0 is the real-time acquisition of data from the factory floor which can lead to immediate improvements in manufacturing costs and product quality. Next in expectation are operations agility, supply chain costs and product innovation, followed by time-to-market, improved customer satisfaction and revenue increase. Since IIoT and predictive analytics directly affect OEE (Overall Equipment Effectiveness), it turns out that when properly implemented and used, there is a dramatic positive effect on revenue growth, as we will demonstrate in this white paper.
ADOPTION OF INDUSTRY 4.0 EXPECTATIONS AND FACTS
In a 2015 survey by PwC of more than 2,000 manufacturers in 26 countries, one-third had already achieved more than 35% of digitization of their factory floors, and almost 77% is planning to reach a greater than 65% level of digitization by 2020. The reality is however is that this is an optimistic view, based on expectation of favorable consumer sentiment and capital market conditions.
What GEMBO has found is that economic uncertainties dramatically temper the adoption rate for smart manufacturing, because of the high capital and operational expenditures associated with replacement of legacy equipment with the latest and greatest digitized equipment. GEMBO has further found that the majority of today’s factory automation consists of data islands with no means to access the machine data in real-time or at all.
What GEMBO has found is that economic uncertainties dramatically temper the adoption rate for smart manufacturing, because of the high capital and operational expenditures associated with replacement of legacy equipment with the latest and greatest digitized equipment. GEMBO has further found that the majority of today’s factory automation consists of data islands with no means to access the machine data in real-time or at all.
There are two common situations, GEMBO has observed. The first one is the existence of the “Digitally Controlled Data Island” where factory automation installations include a PLC or some other form of digital control, but with no network access. Therefore, once programmed, they perform sensor data acquisition and control as a closed system with no access to real-time sensor data. This precludes such systems from providing data for the enablement of smart manufacturing.
The Second one is that of “Missing Critical Data”, where the PLC only monitors signals that are directly related to particular subsystems of the machine, and in most to all cases, monitors only the actions of the machines, related to total parts, good parts and bad parts, leaving out other variables that can provide imperative complementary insights in the root cause of diminished OEE or that are important in order to be able to make highly accurate predictions when to conduct equipment maintenance. Monitoring vibrations, noise, ambient temperature, humidity and power supply conditions are among just a few of these variables that are largely omitted, but yet can contribute in very important ways. Furthermore, it should be noted here that such incompleteness of data holds equally true for machines that are network-enabled, no matter if they belong to the most recent generation of factory automation equipment.
CONNECTING DATA ISLANDS AND EXTENDING DATA ACQUISITION WITH GEMBO PRECARE
GEMBO has recognized the earlier mentioned practical problems which manufacturers face on an ongoing basis, no matter where they are in their journey to transition to smart manufacturing. Where many excellent products exist today for the back-end processing of machine data, GEMBO is unique in that it also solves the “last mile” problem in addition to the back-end processing for critical KPIs and predictive analytics.
GEMBO Precare Agents deploy their own sensors to complement sensor data, if accessible, from the machine, such as from a PLC, or rely entirely on its own sensors where there is no access at all to sensor data. In either case the agents connect via a TCP/IP infrastructure, Ethernet or WiFi, to a GEMBO Precare Cloud. In this fashion GEMBO Precare connects machines into a network infrastructure and eliminating existing data islands.
GEMBO further distinguishes itself from other IoT solution providers via reliable, industrial grade agent and sensor hardware, as well as its broad and deep knowledge of sensor technology. These hardware components are specified to operate in harsh environmental conditions, commonly encountered in heavy industries manufacturing, such as construction materials manufacturing.
Although there are literally hundreds of temperature probes available for the same temperature measurement range, making a selection just based on temperature measurement range, substance to be probed (i.e., solid, liquid or gas), probe assembly and electrical interface is not sufficient. The table below lists a subset of variables that are critical in the selection of the correct probe for the job.
Parameter | Explanation |
---|---|
Type | Thermocouples are categorized based on their bimetals used. RTDs are categorized based on their resistive material used and impedance. |
Range | The measuring range dictates which category of thermocouple to use. |
Accuracy | Often overlooked whereas a low accuracy can lead to many problems. |
TTC - Thermal time Constant | Often overlooked how fast the probe settles for a temperature step change. |
Power supply | Often overlooked difficult to reach wall outlets. |
Vibration | Often overlooked for use in harsh environments, resulting in failures. |
Shock | Often overlooked for use in harsh environments, resulting in failures. |
GEMBO PRECARE SOLUTIONS
GEMBO Precare uses the power of data, analytics and machine learning to mitigate and minimize the impact of equipment related adverse events, providing the following solutions:
- Predictive maintenance. Avoids costly unscheduled and unnecessary scheduled downtime. Instead predicts when precisely to schedule maintenance.
- Catastrophic failure prevention. Avoids costly catastrophic failures, for instance by early detection of abnormal vibrations in rotating machine parts or due to unbalanced machine parts.
- Maintenance/repair time reduction. Avoids expensive maintenance/repair crew hours with prior knowledge of the kind of maintenance or repair and which spare parts are needed.
- Overall Equipment Effectiveness & customer satisfaction optimization. Maximizes OEE, revenues and margins by eliminating unnecessary and unplanned downtime by keeping any downtime at a minimum, rate of production optimal and quantity of scrapped products low.
PREDICTIVE MAINTENANCE
OEE
Just as predictive maintenance is important to optimize equipment availability and to eliminate unnecessary scheduled downtime, so is OEE for productivity. OEE is an important KPI and tool to discover events and factors that adversely affect equipment effectiveness and customer satisfaction.
OEE is expressed as OEE = availability x performance x quality. Each of these components is measured as follows:
- Availability; measured as the ratio of actual production time and total production time for a given production batch.
- Performance; measured as the ratio of actual quantity produced and quantity of units that could have been produced under ideal condition for a given production batch.
- Quality; measured in terms of the ratio of the quantity of good units and the quantity of total units the machine produced in a production batch.
GEMBO Precare not only collects all data necessary to calculate availability, performance, quality and OEE, but drills down to the cause(s) of diminished OEE. An example is shown below for an installation consisting of two chillers and two condensers serving the building lobby and the cafeteria with one pair of chiller and condenser for each space at ACME CA, USA.
At the top level the OEE KPIs are shown for the complete factory. Each OEE KPI lists also the details. For instance, for the Availability KPI the hours of unplanned, planned and total run time are shown.
Since the availability KPI is relatively low compared to the performance and the quality KPIs, the next step is to find out which equipment is experiencing high levels of downtime. Examining the floor plan reveals that machine 1 on floor 1 is currently in the red zone. This doesn’t mean yet that this machine is responsible for the availability KPI to be so low, since this is just a snapshot at the present moment.
At the same time the bar graph below shows that the main reason for the low availability is due to a relatively high number of assist hours.
Clicking on the assist bar shows that machine 1 on floor 2 contributes to nearly 100% of the assist hours.
Further drill-down from here is possible into the exact reason(s) for the high number of assist hours, depending on the number of drill-down levels for assists configured in GEMBO Precare.
GEMBO PRECARE AGENTS
GEMBO Precare deploys agents which can talk directly to equipment controllers over a host of different physical interfaces and protocols, as well as gather machine data independently from the machine’s controller. The key agent features are listed below:
- Real-time data acquisition. GEMBO Precare agents collect any equipment and ambient vital data in real-time for overall equipment health analysis and trends.
- Any equipment vitals. Whichever is needed to be monitored, including abnormal power supply voltage fluctuations, unusual noise and abnormal vibrations caused by moving parts, ambient temperature, humidity, etc.
- Any connectivity. Equipment vitals may be collected through sensors on GEMBO Precare agents, from the equipment’s own programmable logic controllers or through a combination of both.
- Low latency. GEMBO Precare agents run on microprocessors which reside in the vicinity of the equipment, performing on the spot anomaly detection, machine vision, complex event processing and other latency-sensitive tasks.
- Machine learning. These microprocessors are powerful enough to perform inference, using the machine learning models trained by the GEMBO Precare Cloud platform.
- GEMBO Precare agents connect to your choice of private, public or hybrid Cloud, where the data can be used to train machine learning models, visualize KPIs and other data, issue alarms and periodic reports.
CONCLUSIONS
GEMBO Precare has a set of powerful data acquisition, analytics, predictive and OEE tools for manufacturing equipment. GEMBO Precare compiles critical KPIs that can be used to easily trace back which machine or machine subsystem is responsible for a low KPI score. GEMBO Precare is also able to make predictions at equal or better than humanly possible for machine maintenance to be scheduled before a failure occurs. But most importantly, unlike other market solutions, GEMBO Precare is able to deploy its own sensors independently from any machine controller and fully connect any machine data island to the GEMBO Precare Cloud at a fraction of the cost of a new machine; hence, saving manufacturers from having to make large and risky CAPEX and OPEX investments for new machines.
CONTACT US
The GEMBO Precare IIoT platform consists of GEMBO Precare Edge, GEMBO Precare Cloud and GEMBO Precare KPIs. GEMBO Precare Edge agents are responsible for data acquisition, digital twin representations of the machines, edge processing and communicating machine data to the GEMBO Precare Cloud. GEMBO Precare Cloud uses the machine data for predictive analytics, including machine learning for predictive maintenance, and real-time compilation of critical KPIs, such as equipment Availability, Performance, Quality, OEE, MTBF and MTBA.
Broad and deep knowledge of sensor technology means that GEMBO is able to understand measurement and behavioral parameters which are critical for each machine in the process flow and their relevance to the predictions that can be made from the data. The importance of this cannot be overstated and is best illustrated via the temperature probe example here below.
GEMBO Precare collects failure data and uses machine learning to construct models that can accurately predict when a failure has the highest probability of happening. An example is shown below. GEMBO Precare predictions take scheduling time into consideration. For example, if scheduling maintenance requires 30 days ahead scheduling, the prediction is made for 30 days out. Prediction results are expressed in failure probability and accuracy. GEMBO Precare achieves close to 100% accuracy as shown in the example, where a prediction is made on day 110 when a failure will occur. The actual day of failure is at day 159, whereas GEMBO Precare predicts with a 99.9% probability and 99% accuracy that the failure will happen on day 157.
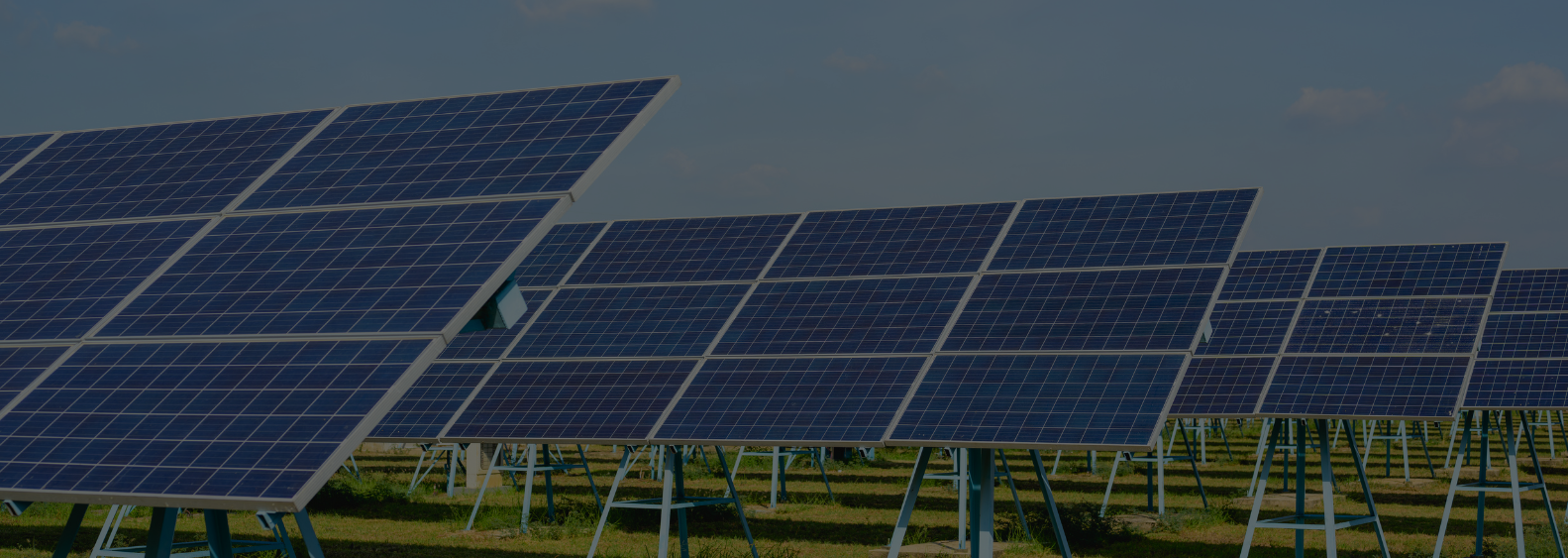
GEMBO ( www.gembo.co ) has a SaaS Industrial IOT Platform which provides enterprises and users with mission critical data, insights and decision making tools enabling cost reduction and revenue growth through optimization via the use of IoT, Artificial Intelligence and Machine Learning. Farm Operator Intro A large-scale solar farm operator, managing a 50 MW solar farm with significant investments in infrastructure. The solar farm has a current efficiency of 24% and a target of increasing this efficiency over time. The farm operates in an area where electricity prices are approximately $32 per MWh, and it has a large operational budget dedicated to maintaining its equipment. GEM has deployed the Precare cloud, predictive maintenance and solar power prediction Package, on the customers Data, extracted from the solar farm's SCADA system. Problem Due to the complexity of solar equipment and environmental factors, the customer has faced a gradual decline in efficiency, dropping from 26% to 24% over the past few years. As a result, the farm's revenue has been impacted by lower power output, and operational expenses have been steadily increasing. Furthermore, the lack of predictive maintenance (PdM) has led to frequent downtime and unplanned maintenance, further increasing the inefficiencies. The farm owner reached out for a solution that could improve operational efficiency, reduce costs, and enhance overall power production by using predictive analytics to forecast and mitigate potential issues before they occur. Solution After evaluating the farm’s needs and existing system, GEM’s Predictive Maintenance (PdM) and Solar Power Prediction packages were discussed and found as the right fit. This solution was implemented through GEM’s Precare analytics platform, which helps monitor and predict performance, detect faults early, and optimize maintenance schedules. The key components of GEM’s solution included: Real-time Monitoring: Continuous monitoring of solar inverters, panels, and environmental conditions. Predictive Maintenance: Advanced machine learning models to predict the likelihood of system failures and performance degradation. Power Output Forecasting: Predictive analytics to model expected energy production based on historical data and real-time inputs, leading to more accurate forecasting and optimizing grid management. Results After deploying GEM’s predictive maintenance and power optimization system, GEM provided the customer a calculation of a 3 year business analysis Financial Benefits: Revenue Increase: The system’s efficiency would increase by an additional $864,320 in revenue over 3 years due to the increased power output from the higher efficiency (25% vs. 24%). OPEX Reduction: Predictive maintenance would reduce the monthly operational expenses by 3%, leading to OPEX savings of $78,810 over the 3-year period. Net Benefits: The customer will realize a total net benefit of $758,130 after deducting the initial system cost and subscription fees. ROI: The ROI of 658.13% was computed from the net benefits of $758,130 after accounting for system cost investments.
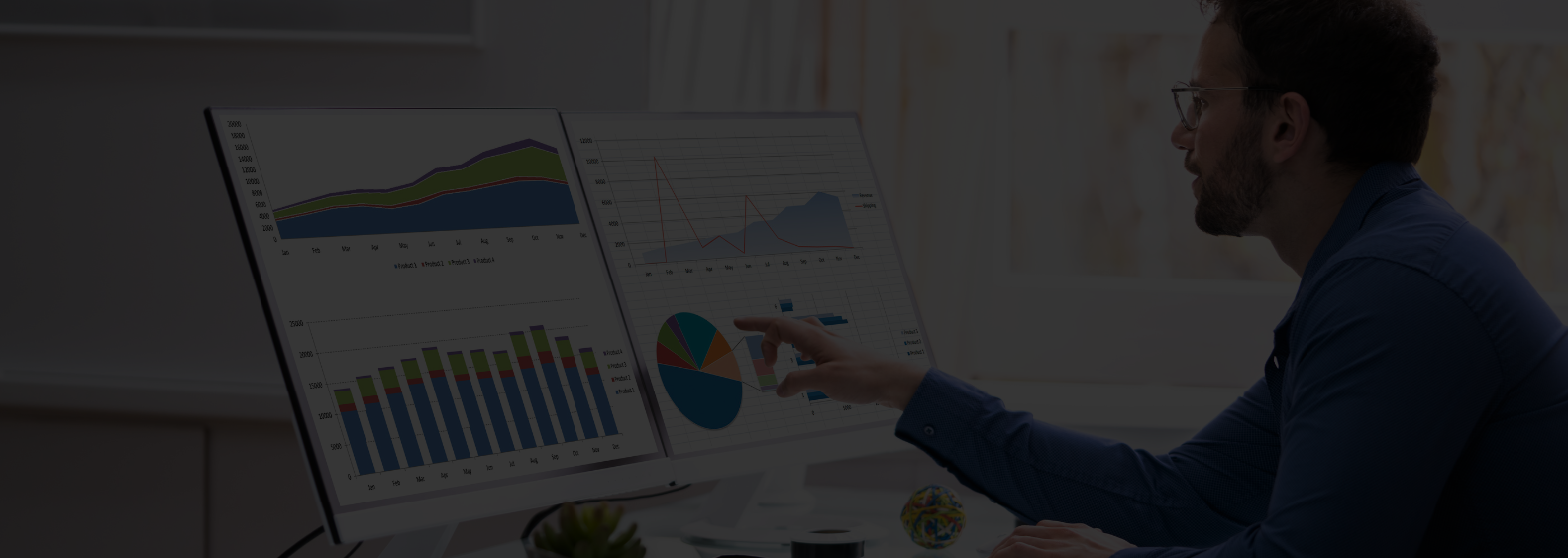
SELF SERVICE DATA STUDIO CASE STUDY This case study showcases GEMBO, a leader in SaaS Industrial IoT Platforms, enhancing a global manufacturer's operations by deploying its Precare Data Studio BI product across 70 machines. The solution, focused on self-service data management and analytics, resulted in significant manpower savings, improved self-service efficiency, and automated analytics distribution. Unique to GEMBO Precare is its ability to deploy independent sensors, connecting any machine to its cloud, thereby offering predictive maintenance and operational insights without substantial capital expenditure, distinguishing it from other market solutions.
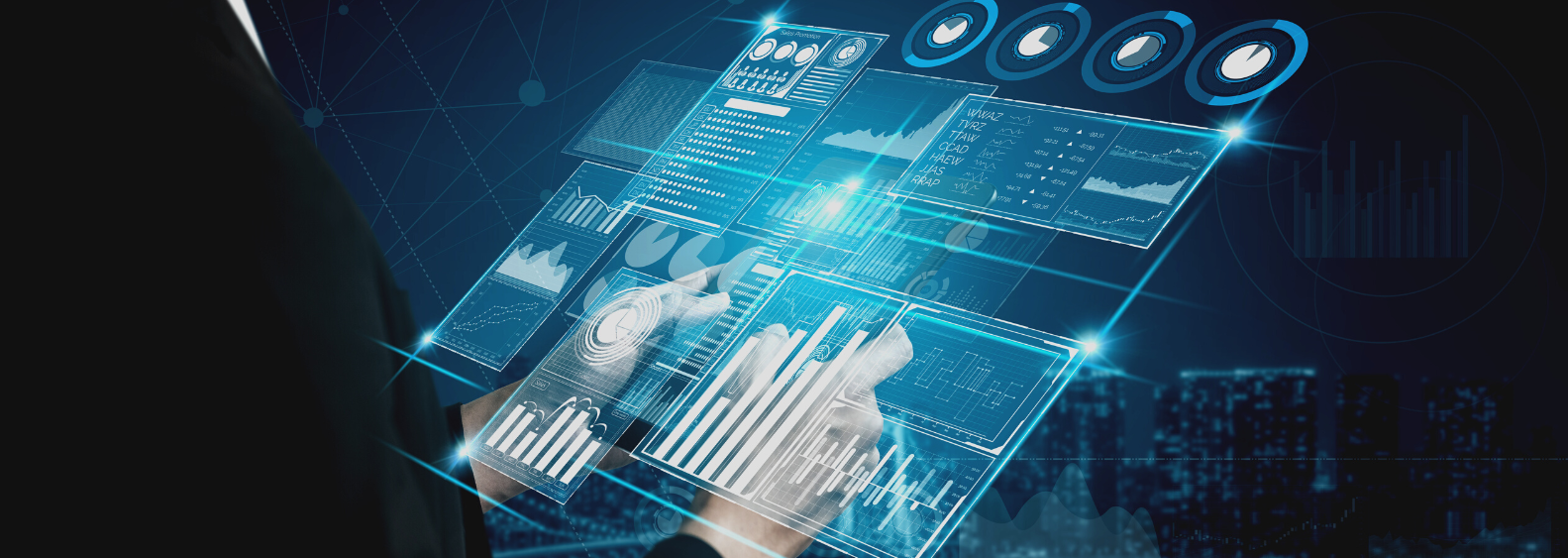
GEMBO ( www.gembo.co ) is a leading innovator in the world of SaaS Industrial IoT Platforms, with a proven track record of delivering cutting-edge solutions that help customers achieve their business goals. The company's platform uses a combination of IoT, machine learning, and AI to provide customers with real-time insights into their operations, enabling them to make better decisions and optimize their performance. GEMBO is committed to continuous innovation, and the company is constantly looking for new ways to use technology to help its customers succeed. Customer Intro The customer is a multi $B Tier one manufacturer, with multiple factories around the globe. Manufacturing lines vary between electronic manufacturing to semiconductor, serving a variety of markets including Automotive, Networking, Industrial, Audio and Gaming. GEM has deployed Precare Cloud , Precare Edge , OEE Availability and predictive analytics Package . Its footprint grew from a few machines to over 90 machines in a few of their factories. Problem The customer has been utilizing OEE Availability analytics to identify machines with low availability and assigning them to responsible technicians. The customer's management team has been overwhelmed with emails from technicians providing status updates on the machines after they have taken corrective actions. The customer intends to streamline the process of monitoring machine status from the receipt of OEE Availability status to the resolution of availability issues, enabling the machines to operate efficiently based on established availability KPIs. The customer has provided the following workflow to illustrate their requirements.
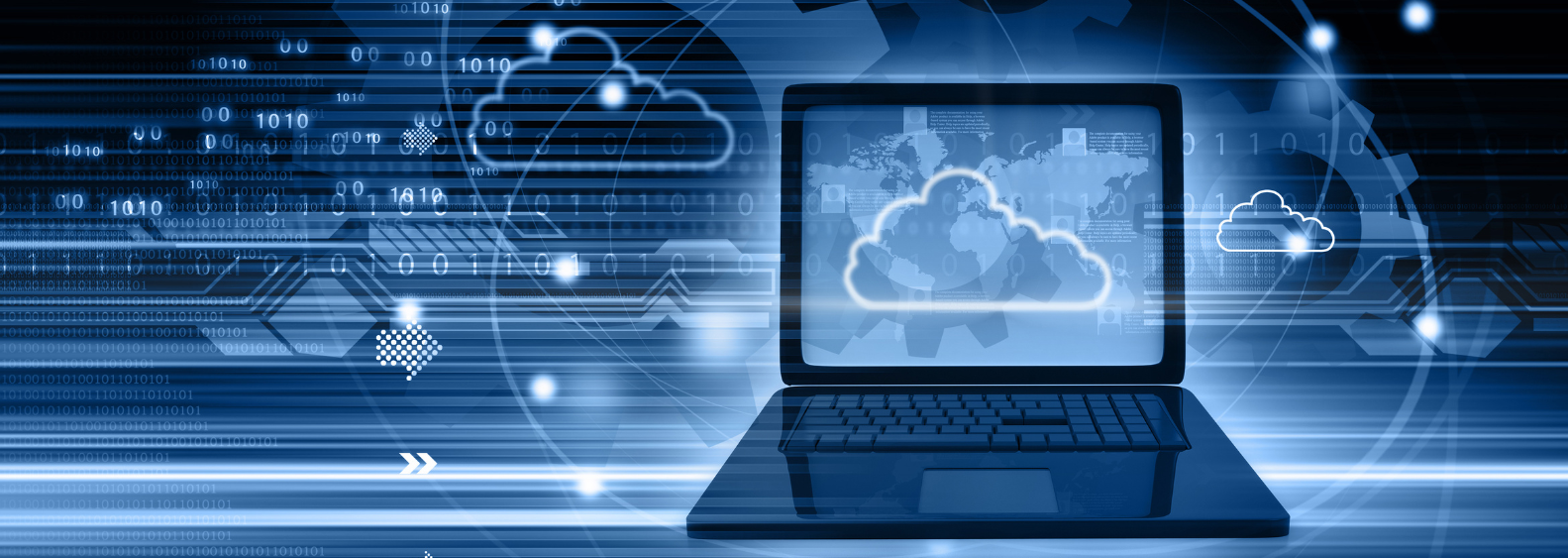
GEMBO ( www.gembo.co ) is a leading innovator in the world of SaaS Industrial IoT Platforms, with a proven track record of delivering cutting-edge solutions that help customers achieve their business goals. The company's platform uses a combination of IoT, machine learning, and AI to provide customers with real-time insights into their operations, enabling them to make better decisions and optimize their performance. GEMBO is committed to continuous innovation, and the company is constantly looking for new ways to use technology to help its customers succeed. Customer Intro The customer is a Tier 1 multi-billion dollar global manufacturing leader in the power, discrete semiconductor, and passive electronic components (PES) space. GEMBO has deployed a suite of innovative, cloud-based solutions to the customer, including Precare Cloud, Precare Edge, OEE Availability, Performance, and Quality packages. These solutions have been deployed to 70 machines across two factory floors, and the customer intends to scale the deployment across their entire Asia-Pacific footprint. Problem The customer's equipment engineering team has been managing the productivity of their machines through manual Excel reports. These reports are prepared by machine operators, checked and consolidated by supervisors, and then reported to management by factory floor managers. The customer needs to minimize or eliminate these manual reports to improve the accuracy of the reports and save on manpower costs. The following are examples of manual reports that the customer needs to eliminate: 1. Calculation of the share of subcategories of Performance and Quality 2. Calculation of the percentage of Planned Downtime to Total Run Time 3. Graphical presentation of OEE Availability with Time Frame These reports are time-consuming and error-prone. They also require a significant amount of manpower to create and maintain. By eliminating these manual reports, the customer can improve the accuracy of their productivity data and save on manpower costs. Solution After a technical discussion with the customer, GEM integrated the manual Excel reports into the OEE analytics framework. The team then implemented the following process: Define the customer requirements Gather the excel reports Create an output image showing changes in the analytics Define data to be collected Collect data from the customers Collect the data from customer Validate the completeness of the data based on the requirements Collect missing data Confirm with customer if all data are accurate and structured properly based on their requirements Prepare the design Overview Customer Requirements Use Case System Diagram Code Diagram Review and approval of the design Testing on Developer Environment Deployment to Production Customer acceptance Results The migration of previously manually prepared OEE reports to a digital format using OEE Analytics resulted in the following benefits: 100% savings on manpower costs, as the reports are now generated automatically and do not require manual input. 100% accuracy, as the data is collected directly from the machines and is not subject to human error. 100% time savings for management, as they no longer need to spend time reviewing and validating the reports. Improved decision-making, as the Operations Group can now access real-time data and insights to make better decisions. Increased efficiency, as the Operations Group can now focus on core tasks and not on data entry and reporting. Conclusions GEMBO Precare has a set of powerful data acquisition, analytics, predictive and OEE tools for manufacturing equipment. GEMBO Precare compiles critical KPIs that can be used to easily trace back which machine or machine subsystem is responsible for a low KPI score. GEMBO Precare is also able to make predictions at equal or better than humanly possible for machine maintenance to be scheduled before a failure occurs. But most importantly, unlike other market solutions, GEMBO Precare is able to deploy its own sensors independently from any machine controller and fully connect any machine data island to the GEMBO Precare Cloud at a fraction of the cost of a new machine; hence, saving manufacturers from having to make large and risky CAPEX and OPEX investments for new machines. Contact Us Visit us at www.gembo.co or contact us via email at sales@gembo.co