Predictive Maintenance: what it is and how is it transforming manufacturing?
Predictive maintenance uses real-time data collected via the Industrial Internet of Things (IIoT) platforms to continuously analyze the machine's condition as it operates normally so that any sudden and unexpected failure can be avoided. It allows organizations to monitor and check the status of indicators like lubricants, speed, and bearing speed. These tools detect any abnormal behavior when the machine is operating normally and immediately send the report to the owner of the machine so that any potential incident can be avoided. The body content of your post goes here. To edit this text, click on it and delete this default text and start typing your own or paste your own from a different source.
In simpler terms, predictive maintenance analyzes and monitors the health of an asset in real-time and reports abnormalities to the owner in real-time to avoid any potential failure, breakdowns, and so on. It also offers the manufacturer an option to plan maintenance as per their production schedule. agraph
Working of predictive maintenance
Predictive maintenance works in the following ways:
- Digital status analytics: Mining of machine digital repositories, understanding the meaning of statuses, and using those together with Machine learning, digital rein, and Artificial Intelligence to predict specific failures. This is specificity important for more expensive, critical, and complex machines.
- Infrared analysis: Infrared analysis uses the temperature of the machine as an indicator of the problem. Abnormalities related to airflow, cooling, and motor stress can be identified using this method.
- Vibration analysis: Vibration analysis is one of the most common predictive maintenance methods used in plants with rotating machinery. Abnormalities related to balance, alignment, loose parts, etc can be detected via this method.
- Sonic acoustical analysis: This method uses sound converted into auditory or visual signals to detect problems like under-lubrication in both low and high rotating machinery.
How is predictive maintenance different from preventive maintenance?
- Preventive maintenance:
It occurs at regular time intervals depending on the lifecycle of the machine, irrespective of its usage, to prevent any issues.
- Predictive maintenance:
It occurs only as needed based on the reports provided by the IoT sensors regarding the status of the machine. This allows manufacturers to schedule maintenance to avoid any sudden unexpected potential breakdowns or failures.
How is predictive maintenance different from condition-based maintenance?
- Condition-based maintenance
This type of proactive maintenance uses sensors to collect real-time insights about a piece of machine based on various factors like temperature, vibration, and pressure. In this case, the service is only deployed when the machine conditions demand. This type of maintenance includes a risk of multiple machines needing service simultaneously.
- Predictive maintenance
Predictive maintenance is a type of condition-based maintenance, but it uses IIoT sensors at a larger scale to gain continuous insights into the machine's condition. Along with the equipment condition insights, it also uses big data methodologies to predict the machine degradation depending on the history of the equipment. It allows technicians to detect potential issues beforehand to avoid any potential issues and schedule maintenance more efficiently.
Key benefits of predictive maintenance
Here are the top five benefits predictive maintenance offers your organization:
- Minimized downtime
Predictive maintenance alerts technicians of any potential issues in advance so that they can take action upon them beforehand and avoid any unexpected failure of breakdowns. This reduces the downtime by as much as 30%, avoids disruptions that damage brand reputation, and allows you to schedule multiple service procedures.
- Better productivity
As there are minimal disruptions due to unexpected malfunction or breakdown, the productivity is optimized. The service time-to-resolution is 83% faster, no productive lags are there, maximum uptime is achieved, and asset usage improves.
- Lower field service costs
Pre-planned maintenance saves major costs to service departments by minimizing service truck rolls, better first-time fix rates, and optimized maintenance costs.
- Improved product design
The equipment data collected via the IIoT sensors allows product designers to increase equipment life spans, enhance product durability and reliability, and build more efficient machines in the future.
- Enhanced worker safety
By predicting and resolving the chances of an unexpected potential failure, you can ensure that no employees are working near machinery that is at risk of a potential malfunction. The service to the machine can be provided before it becomes hazardous..
Implementing predictive maintenance
Follow these steps to start with your predictive maintenance. There are several ways to do it:
Option 1: Do it yourself:
Program design
- Acquire permissions from your senior management
- Outline the advantages of the predictive maintenance
- List out equipment with a history of high failure rates along with its causes
IIoT installation
You can use machines having sensors, connected to an IIoT platform to conduct predictive maintenance.
System integration
Use IIoT tools to implement predictive maintenance. The connectivity established by IIoT based condition monitoring can be used to enhance efficiency using analytics, automation, and integration between OT and IT.
Schedule maintenance
Your team is equipped with advanced real-time alerts about equipment insights for scheduling service delivery and coordinating maintenance.
Option 2: accelerate with the combined platform and data science:
- Factory and machine survey: analyze all sources of data and identify critical ones
- Onboard machines: use iiot platform with already support for the functionality: from data collection to prediction.
- Focus on what’s important: data science and modeling: Avoid expensive timely and risky data integration: the platform will make data concerted for information to your data scientist or subject matter expert.
- Deploy and periodically train: deploy the model and utilize the platform user interface, alarms, and dashboard to manage your predictive Maintenance operations.
Use predictive maintenance and stand apart from the competition
The ultimate and numerous benefits of
predictive maintenance will provide you an edge over your competitors. Don’t face the risk of downtime and its associated hurdles. Be proactive with your plan and service delivery using predictive maintenance.
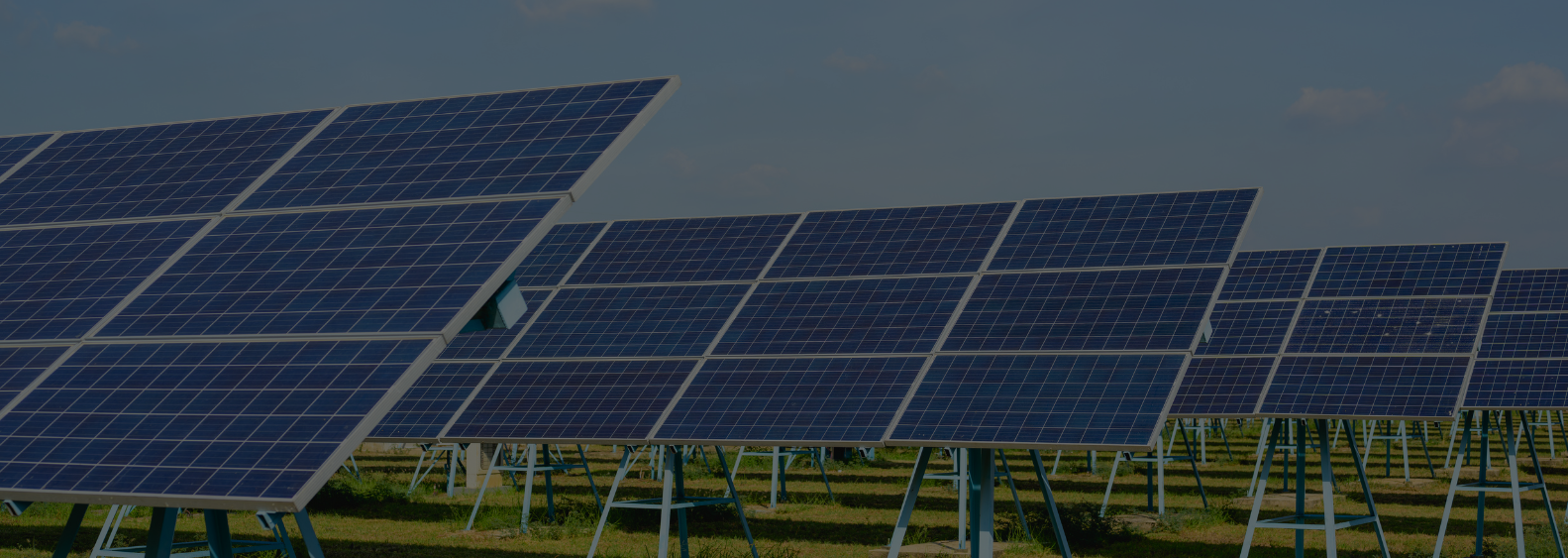
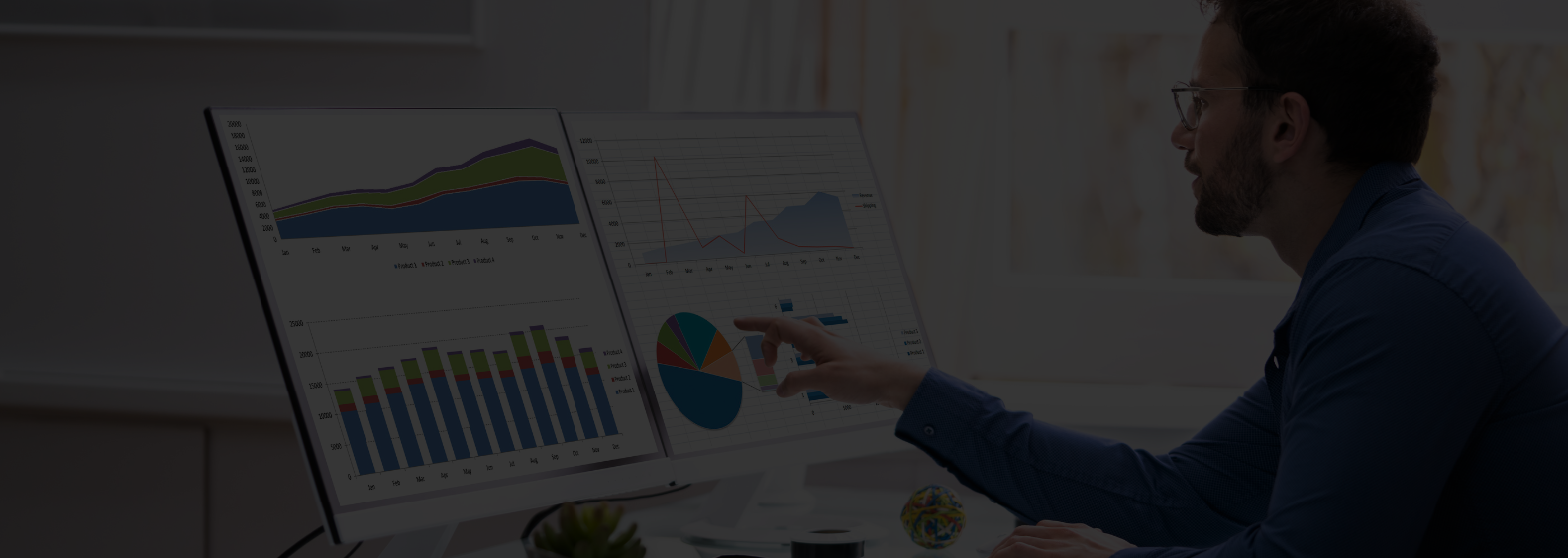
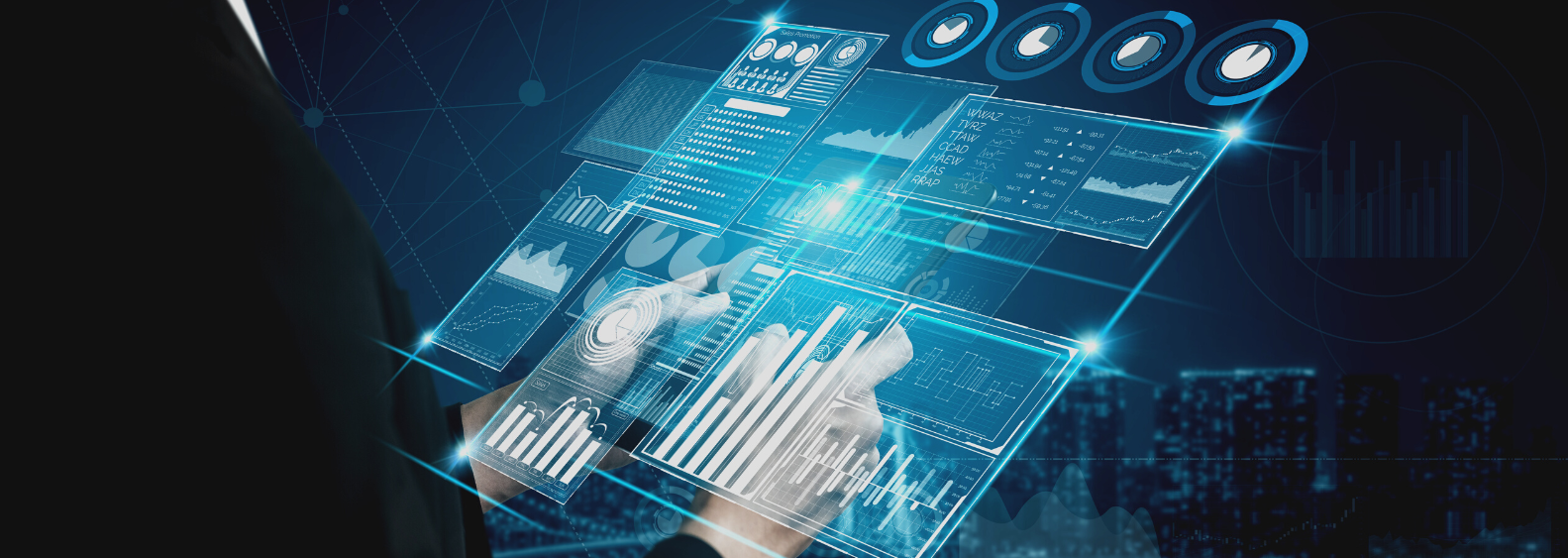
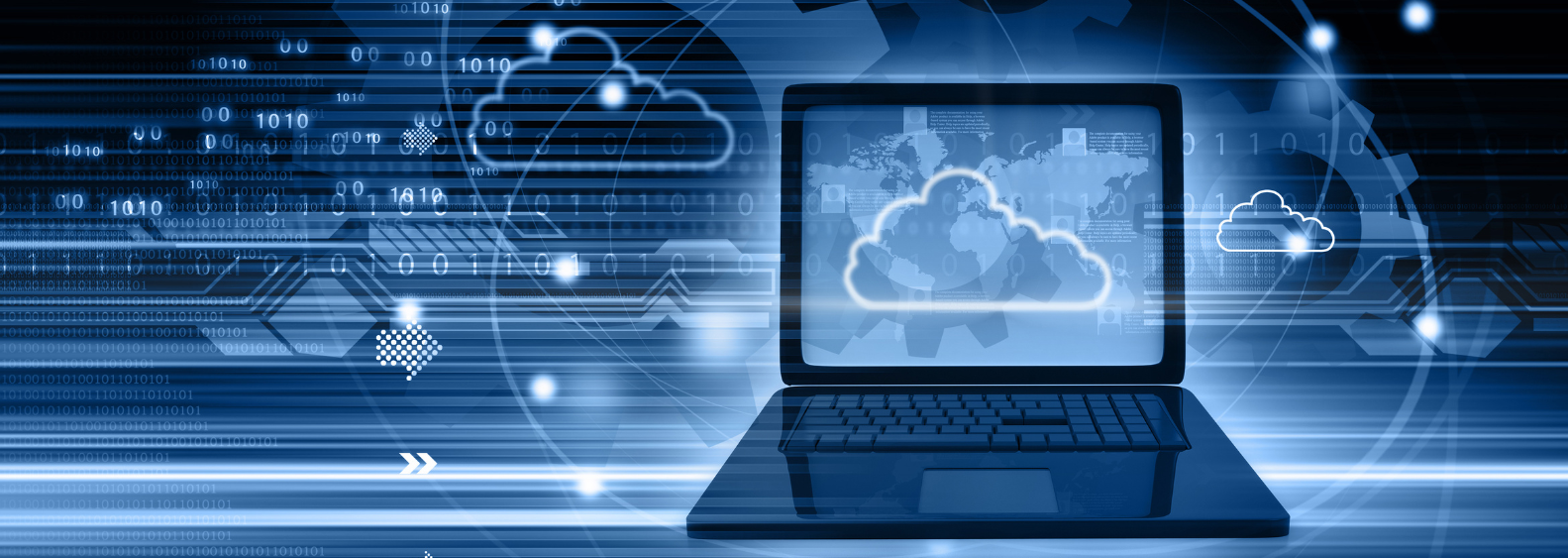
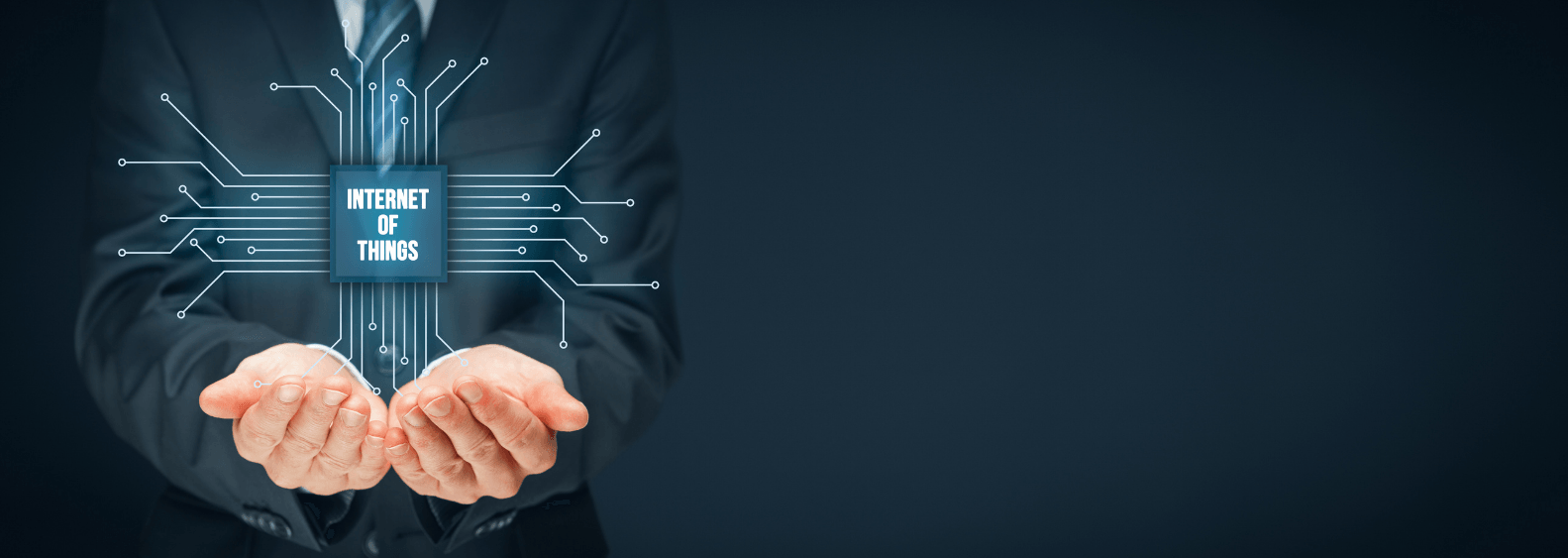